The Reality of Generative AI: How Poor Data Practices Are The Real Roadblock to Digital Transformation
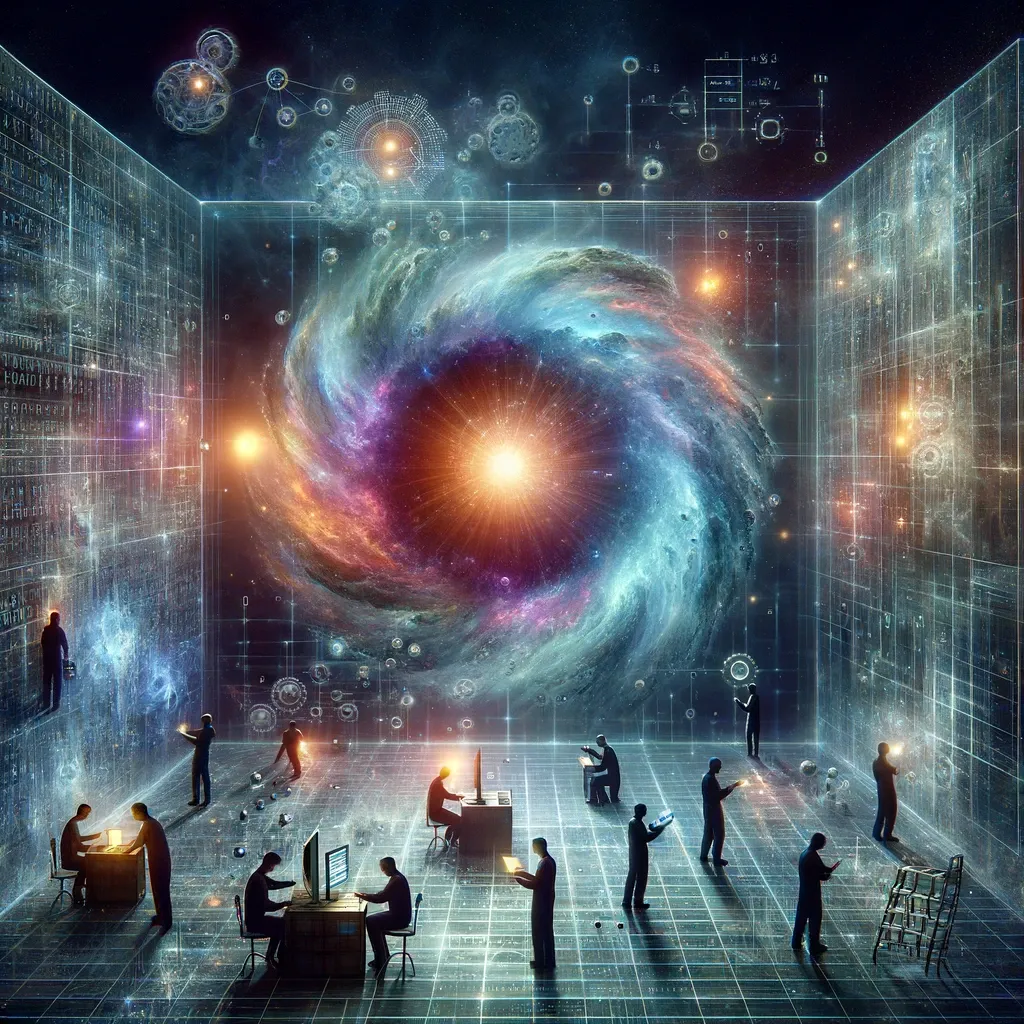
Introduction
In today's rapidly evolving digital landscape, the buzz around Generative AI is impossible to ignore. From creating stunning visual art to revolutionizing natural language processing, its capabilities are capturing the imagination of the tech world. This groundbreaking technology represents not just a leap forward in machine learning, but a potential paradigm shift in how businesses interact with data and technology.
However, amidst this excitement, there lies a critical, often-overlooked factor that determines the success of any AI-driven initiative: the state of a business's data practices. While the allure of cutting-edge solutions like Generative AI is undeniable, they are merely the tip of the iceberg in the vast ocean of digital transformation. Beneath the surface lies the true challenge that many businesses face: outdated, inefficient, and often problematic data management practices.
This blog post aims to shift the focus from the glittering promise of new technologies to the foundational elements that truly drive digital transformation. We will explore why addressing and rectifying bad data practices is not just necessary for harnessing the power of Generative AI, but is essential for any form of meaningful digital progress. As we delve deeper, we will uncover how businesses, blinded by the allure of new tech, often overlook the fundamental data issues that hold them back, not just in the realm of AI, but in all aspects of digital evolution.
The State of Data in Businesses Today
In the contemporary business landscape, data is often likened to a new form of currency or oil, underpinning the most successful digital transformations. However, the reality for many businesses is a far cry from this ideal. Data is frequently mishandled, mismanaged, or simply misunderstood. This mismanagement manifests in various forms, from siloed data repositories that impede access and collaboration to inconsistent data formats that hinder analysis and application.
Siloed data, in particular, presents a significant challenge. These silos emerge over time due to departmental divides, legacy systems, and the absence of a unified data strategy. They not only create barriers to information access within an organization, but also lead to duplication of efforts and inconsistencies in data. Such an environment is antithetical to the agile, data-driven decision-making that modern businesses require.
Furthermore, the issue of data quality cannot be overstated. In many cases, businesses are plagued by outdated, inaccurate, or incomplete data. The implications of this are twofold: first, it directly impacts the effectiveness of decision-making processes, and second, it severely limits the potential of advanced data analytics and AI/ML applications. Without clean, accurate, and well-structured data, the most advanced AI algorithms will fail to deliver meaningful or reliable results.
Lastly, the lack of robust data governance and security practices poses a significant risk. In an era where data breaches and privacy concerns are increasingly common, proper governance is not just a regulatory requirement, but a critical component of customer trust and business reputation. Effective data governance ensures that data is not only secure, but also utilized in a manner that is ethical, compliant, and aligned with business objectives.
The worst part about this is that you are very likely nodding your head and aware of these issues in your business right now, but have limited ideas how to change or overcome these issues.
The Illusion of Technological Solutions
In the quest for digital excellence, many businesses are captivated by the allure of new technologies like Generative AI. This fascination, however, often leads to a common pitfall: the belief that technology alone can spearhead digital transformation. While these advanced tools offer substantial capabilities, their effectiveness is significantly hampered when foundational data issues, such as siloed and poor-quality data, are ignored. The misconception that technology can independently resolve these deep-rooted data challenges diverts attention and resources from the critical task of establishing a robust data foundation.
This overemphasis on technology creates a mirage of progress, masking the underlying data inadequacies that hinder real transformation. Businesses investing heavily in AI/ML solutions without addressing their data practices are likely to encounter disappointments. AI initiatives, no matter how sophisticated, cannot compensate for systemic data problems like inconsistency, inaccessibility, and poor governance. These are not mere technical issues, but strategic ones that require comprehensive management and organizational change.
The path to genuine digital transformation, therefore, is not solely through the acquisition of the latest AI tools, but through a balanced approach that prioritizes data management reform. Recognizing that technological advancements, including Generative AI, are most powerful when built upon a solid data infrastructure is crucial. Only then can businesses harness the full potential of digital innovation.
Unlocking True Potential with Solid Data Foundations
The cornerstone of any successful digital transformation lies in solid data foundations. This begins with the recognition that data is more than just a collection of numbers and facts; it's the lifeblood of modern business decision-making and innovation. To truly unlock the potential of technologies like Generative AI, companies must first address the fundamental issues of data cleanliness, availability, and governance. This involves a meticulous process of tearing down data silos, ensuring data accuracy and consistency, and establishing a single source of truth for critical data elements.
Effective data management goes beyond mere organization and storage. It requires a strategic approach to making data accessible and useful. Implementing modern data access methods such as GraphQL and APIs is essential in creating a data ecosystem that is both flexible and secure. These technologies enable seamless integration and utilization of data across various platforms, paving the way for advanced AI/ML applications. Moreover, this approach ensures that data is not only available but also delivered in a format that is immediately actionable.
Data governance plays a pivotal role in this landscape. It's about establishing clear policies and practices to ensure data security, compliance, and ethical usage. As businesses integrate AI/ML into their operations, maintaining control over their data, intellectual property, and trade secrets becomes paramount. Robust governance frameworks provide the necessary oversight to prevent data misuse and ensure transparency, which is crucial for building trust in AI-driven processes.
Finally, the journey towards solid data foundations must be viewed as a continuous process rather than a one-time initiative. It involves regular assessments and updates to keep pace with evolving technologies and business needs. By focusing on these foundational elements, businesses not only prepare themselves for the current wave of Generative AI but also future-proof their operations for emerging technologies.
The Pragmatic Approach to Data Transformation
At the heart of the pragmatic approach to data transformation lies a deep understanding that effective data management is the cornerstone of digital innovation. Many businesses recognize that the journey towards AI and ML integration, especially in the realm of Generative AI, requires more than just technological prowess; it necessitates a fundamental shift in how businesses approach and handle their data. This philosophy aligns with the ethos of many companies, which emphasize not just the adoption of new technologies but the creation of a sustainable and robust data ecosystem.
A pragmatic approach to data transformation focuses on dismantling data silos and fostering a culture of data accessibility across organizations. By employing cutting-edge tools and methodologies, businesses can unify their data landscapes, ensuring seamless data flow and interoperability. This approach not only enhances efficiency but also paves the way for more advanced data analytics and AI applications. The expertise of these companies in crafting bespoke solutions ensures that each client’s unique data challenges are addressed, leading to a tailored path towards digital transformation.
Quality and integrity of data are paramount in any data transformation framework. Companies employ rigorous data cleaning, validation, and management practices to ensure that the data is not just accessible but also reliable and accurate. This commitment to data quality is crucial for the success of AI/ML initiatives, as it guarantees that the insights and outputs derived from these technologies are based on solid, trustworthy data. A data-driven approach ensures that data is a true asset to the organization, enabling informed decision-making and innovation.
Finally, businesses should place a strong emphasis on data governance and security, recognizing these as vital to maintaining customer trust and complying with regulatory standards. Robust governance models ensure ethical and secure use of data. This approach is particularly important in the context of AI/ML, where concerns around data privacy and ethical use are paramount. A commitment to these principles demonstrates an understanding that secure and responsible data management is not just a compliance issue, but a core business imperative.
Conclusion
In conclusion, while the advancements in AI and ML, particularly Generative AI, offer exciting new frontiers for businesses, it's crucial to recognize that the real enabler of these technologies is a solid foundation in data management. A pragmatic approach emphasizes that resolving core data issues is not just a prerequisite for leveraging new technologies but is essential for any form of sustainable digital transformation. Businesses need to shift their focus from merely chasing technological trends to building a robust data infrastructure, thereby ensuring they are well-positioned to capitalize on current and future digital opportunities.
The journey of Data Transformation is not just about adopting cutting-edge technology; it's about transforming the way businesses handle and perceive data. By addressing the fundamental challenges of data accessibility, quality, governance, and security, a pragmatic approach empowers organizations to not only embrace the potentials of Generative AI but to thrive in an increasingly data-driven world.
Let's Make This Real For You
If you're looking to explore how these concepts and technologies can be applied to your organization, reach out to Chris Williams, the maker of improbable things and author of this article. Chris can provide the insights and expertise you need to turn the improbable into the achievable and propel your organization into the future.